16–19 November
The ISPOR Europe 2020 conference was the society’s first ever virtual meeting. It brought together a vibrant community of experts and thought leaders to help to shape the future of health economics and outcomes research (HEOR). The theme of the 2020 meeting was Improving Health: Establishing Incentives and Sharing Value, and it sought to question the fundamental conventions around value, risk, rewards, and the role of public and private sectors.
Reimbursement in challenging circumstances
A Novartis-sponsored educational symposium entitled Gene Therapies – Is Europe Ready to Value and Reimburse the Next Medical Frontier? focused on the challenges of assessing the value of gene therapies against the value of conventional therapies. A fundamental difference between the two is that the costs of gene therapy are incurred all at once, with a single treatment administration that aims to treat the patient for a lifetime, whereas conventional therapies will have continuous costs over time. How should the costs of gene therapy be distributed over time? The solution, according to speaker Professor von der Schulenburg (Leibniz University Hannover), requires novel financing approaches, especially given the uncertainty of the efficacy of gene therapies in the long term. Novel approaches include ‘pay for performance’, where payments are made based on clinical outcomes achieved. Determining the appropriate outcome goals will be helped by strengthening clinical trial evidence during the development planning phase. Strengthening trial evidence will also contribute greatly to the value assessment of the therapy. Decision-makers at this stage could benefit from a valuable tool to aid their assessment: Professor Drummond (University of York) presented a checklist for assessing gene therapies. This included various considerations for the clinical trial evidence, such as whether surrogate endpoints were used and if historical or parallel control data were available. Overall, speakers were enthusiastic about overcoming assessment challenges to ensure gene therapies become available to the patients who need them.
A plenary session entitled Much Ado About Little – Dealing with Limited RCT Evidence for Early HTA and Reimbursement Decisions heard presentations from Professor Sylwia Bujkiewicz (University of Leicester), Professor Eva Susanne Dietrich (Institute for Evidence-Based Positioning in the Healthcare Sector, Germany), Professor Stephen Palmer (Centre for Health Economics, University of York) and Professor Uwe Siebert (Harvard Chan School of Public Health). Expedited approvals for high-priority diseases allow treatments to get to patients faster; however, the level of evidence obtained over a shortened time period, or with fewer patients, may be less strong as a result. This plenary session discussed the challenges of having limited evidence for health technology assessments. Speakers discussed the use of surrogate endpoints; for example, time could be saved by substituting 1-year progression-free survival in place of 2-year overall survival. Professor Palmer spoke of the important paradigm shift that took place when a cancer drug was approved based on biomarker results alone for the first time, rather than based on outcomes directly related to the tumour site. This could lead to ‘basket’ trials, which benefit from increased power by pooling study populations of different tumour types, but which require statistical approaches to account for these differences. Also discussed was the use of causal inference frameworks in the absence of data from randomized controlled trials. Such approaches are welcomed to strengthen evidence, although Professor Dietrich reminded attendees that the decision-makers must understand these methodologies in order to make their assessment.
Patient engagement and healthcare decision-making
Patient and Public Involvement in Healthcare Decision-Making – Are We Maximizing Opportunities? was the question discussed at the second plenary session of Virtual ISPOR Europe 2020. Moderator Bettina Ryll (founder of the Melanoma Patient Network Europe) described her personal experiences, as a physician, of the systemic failures she encountered when her husband developed melanoma. She outlined the need for new thinking and more holistic approaches that consider what patients really want. Axel Mühlbacher (Hochschule Neubrandenburg) went on to describe the methods for assessing patient preferences and priorities to support understanding and value judgements in this area, noting that, “without data, you are just another person with an opinion.”
Advances in patient engagement were described by Yann LeCam (CEO of EURORDIS-Rare Diseases Europe), specifically those seen during his time as an advocate, although he noted outstanding challenges and, in some countries in Europe, even resistance. He highlighted the importance of investing in training advocates to ensure they are well prepared to contribute effectively to specific discussions and expressed hope for a move towards involvement of patients as full partners in healthcare decision-making. Reflecting on this, the increasing and varied role patients have played in informing decisions of the European Medicines Agency over the last 25 years was outlined by Nathalie Bere of the European Medicines Agency.
Summarizing, Dr Ryll noted that patient priorities overlap with those of other stakeholders, sometimes pharmaceutical companies and payers, but they represent a very important and independent viewpoint, and she further emphasized the benefit of providing data to support their testimonies.
Cost-effectiveness
Darius Lakdawalla (University of Southern California) and Charles Phelps (University of Rochester) presented their thoughts on Aligning Cost-Effectiveness Analysis (CEA) Methods with Real-World Patient Preferences. Noting the limitations of traditional CEA for healthcare interventions, such as elements of value that are excluded from consideration and the context-specific value of a quality-adjusted life-year (QALY), they proposed a new approach, termed ‘GRACE’, which employs a Risk-Aversion and Severity-Adjusted willingness to pay threshold and a Generalized Risk-Adjusted QALY. Professor Phelps suggested the use of GRACE rather than traditional approaches “when you want correct answers” to cost-effectiveness questions.
Machine learning
The Introduction to Machine Learning for Health Economic and Outcomes Research workshop was presented by Turgay Ayer (Georgia Tech), Jag Chhatwal (Harvard) and Selin Merdan (Value Analytics Labs) and sought to introduce the current landscape in HEOR through the lens of state-of-the-art modern machine learning (ML) techniques. In particular, they reviewed basics and key concepts of modern ML techniques for descriptive, predictive and prescriptive analytics. Data transformation in the HEOR space has been dramatic – real-world evidence (which depends on big data/data science) does not even appear in the top 10 topics for ISPOR in 2018; in 2019, it was number 3 and in 2020 it is top of the list. ML can often be confused with statistical modelling, but they are different. Statistical models aim to understand and to explain relationships in an existing/current data set, while ML studies focus on external or unseen data. Generalizability is not an important factor in statistical models, but plays an important role in ML.
There are a number of commonly used ML approaches: linear regression, logistic regression, support vector machines, decision trees, neural networks and random forests. Examples of ML uses in HEOR include identifying risk factors and clustering, estimating treatment effects, identifying variation among providers, classification and prediction of categorical responses, optimal treatment pathways, precision medicine, risk-based cost-effective modelling, patient similarity analysis and clustering, and creating niche market access strategies by identifying relevant patient populations.
Attendees heard from Jag Chhatwal on emerging applications and future directions in ML, which covered the increased use of smartphones and wearables. For example, the Apple Heart Study looked at detection of arterial fibrillation through Apple Watch – this may have a huge impact if it were to be cost-effective and if it led to a reduction in mortality.
Key takeaway messages from this workshop were that big data are becoming increasingly available and expectations from payers are changing in the era of big data and ML. It is felt that big data could influence the HEOR space and that ML tools could be instrumental in processing big data. There are some successful examples already available, but the large influence of ML on HEOR is yet to be seen.
A podium session on the theme of ML and artificial intelligence (AI) to improve decision-making in healthcare included a presentation from Seye Abogunrin (Roche) who asked the question, ‘Do machines perform better than humans at systematic reviews of published literature?’ ML methods (MLM) were employed for a systematic literature review (SLR) in prostate cancer (mCRPC) that compared the use of a human versus a pre-trained data-mining model and an AI tool (support vector machines applied to textual data). Human time burden was approximately 85 hours, versus 25 hours for each MLM. Although ~15% of records were wrongly or not classified by the MLMs, overall, using these techniques could yield time savings of 70% in abstract screening but “it is important for a human to see a record before it is assigned a final status – we propose having a machine to review alongside a human.”
On a similar theme, Tove Holm-Larsen (Pharma Evidence) discussed conducting meta-analysis powered by AI. The Silvi system was described; this is an automated meta-analysis system that uses AI to enable faster and fully updated meta-analysis. Evidence suggests that the time taken to carry out an SLR/meta-analysis (typically 3 to 6 months) through ML can be reduced using AI. These methods can lower the burden on the human reviewer: the machine can apply search strings across multiple literature sources, and keyword screening allows the machine to screen about 80% of records successfully and can employ different AI techniques and apply training data sets. It is also possible for automated alerts to be set up to incorporate new papers on PubMed to update the meta-analysis automatically.
An interesting talk was given by Simon Renner (Kap•Code), who introduced the concept of using ML in the detection of quality of life (QoL) impact in health-related messages on social media. Patients and caregivers make extensive use of social media, sharing their experiences, difficulties and unmet needs. This represents a large amount of real-world data and real-life testimonies on the patient journey and on QoL. An ML algorithm was constructed to detect impact on QoL and to classify the impacts into five dimensions (adapted from EQ-5D and SF-36). This algorithm has demonstrated that ML and AI are able to detect QoL impact on patients’ testimonies posted on social media. This could serve as a complementary approach to assessing a patient’s QoL. Potential uses include identifying new QoL impacts not covered by patient-reported outcomes or focus groups, adding an additional dimension of QoL and unmet needs to address in a patient support programme, and assisting in real-time decision-making for patient care.
Of considerable interest in relation to the current COVID-19 pandemic,the application of ML models to evaluate COVID-19-related intensive care unit (ICU) utilization in a US population was outlined by Zeynep Icten (Panalgo). This could support COVID-19 care management by analysing trends from large electronic health record (EHR) data sets. Using the Optum de-identified EHR data set, the sample included adult non-deceased patients with a positive COVID-19 test who had been admitted to ICU. Three different sets of features were evaluated: (1) demographics and co-morbidities; (2) plus laboratory measures; (3) plus laboratory measures and vital signs. All ML analyses were conducted using the Instant Health Data platform that established Random Forest as the best ML model and enabled the identification of the top 30 significant features for ICU utilization in the COVID-19 positive population:
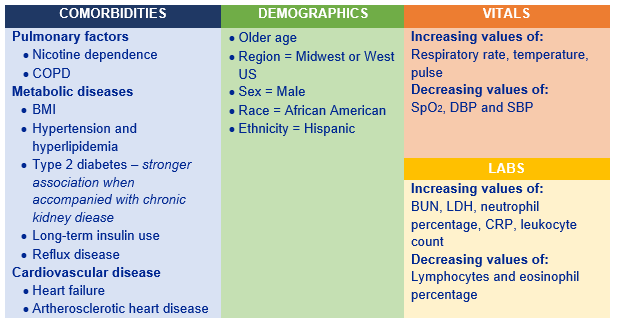
ISPOR has a ‘Machine Learning Methods in HEOR’ Task Force to establish guidance for emerging good practices in the application of ML methodology to traditional ISPOR methods, including economic evaluation, decision sciences and outcomes research in order to improve the value of healthcare delivery.
Overall, the first virtual ISPOR Europe meeting was a great success. Through this conference, HEOR experts and thought leaders were able to discuss with a global audience how to establish, to incentivize, and to share value sustainably for health systems, patients and technology developers. The fundamental conventions around value, risk, rewards, and the role of public and private sectors to drive innovation, investments and to extend progress to broader public health concerns were explored, and the most advanced knowledge in these areas was shared.